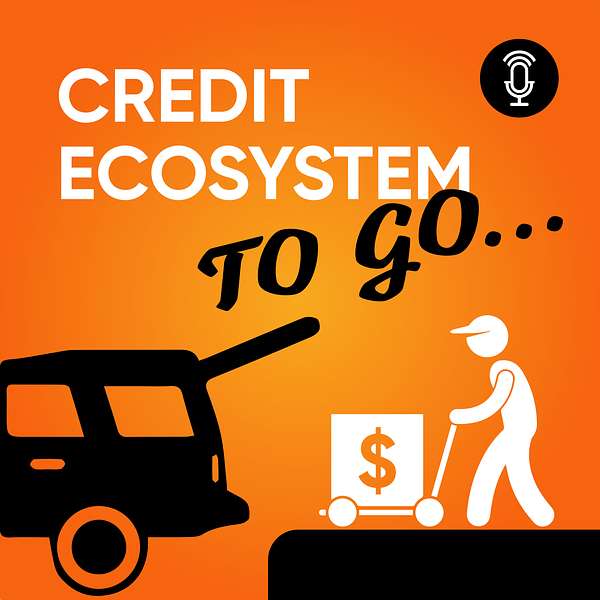
Credit Eco To Go
Credit Eco To Go
Fairness-as-a-Service
Ensuring #fairness at all levels in financial services is the new norm. But how do you do it and how do you know when it is achieved? Kareem Saleh, CEO and Founder of FairPlay AI stops by #CreditEcoToGo to talk about his platform and new #ai fairness methodologies, which do a better job in assessing risk, while at the same time expanding opportunities for credit access. Kareem tells us that “fairness through awareness” and machine-learning algorithms always need to be challenged and refined. Rather than settling for one result, Fairplay exposes their algorithms during model development for alternative outcomes that might not have been well-represented in the first round of data, making the data more sensitive to disadvantaged groups. Kareem hopes this philosophy will enable all financial service entities within the credit cycle, to continue to harness data for the benefit of all consumers. #financialservice #equalaccess #fintech
DISCLAIMER – No information contained in this Podcast or on this Website shall constitute financial, investment, legal and/or other professional advice and that no professional relationship of any kind is created between you and podcast host, the guests or Clark Hill PLC. You are urged to speak with your financial, investment, or legal advisors before making any investment or legal decisions.
DISCLAIMER – No information contained in this Podcast or on this Website shall constitute financial, investment, legal and/or other professional advice and that no professional relationship of any kind is created between you and podcast host, the guests or Clark Hill PLC. You are urged to speak with your financial, investment, or legal advisors before making any investment or legal decisions.
Hello and welcome to another episode of Clark Hill's Credit Ego to Go, curbside thought leadership for financial services. My name is Joanne Needleman and I am a partner at Clark Hill as well as a member of the firm's banking and financial services practice group. Today we're going to talk about fairness. It is the new buzzword for financial services, um, but I always ask myself, what does fairness look like? And more importantly, how do you know when you achieve it? So my guest today is going to help me explore this topic. Kareem Saleh is the founder and CEO of FairPlay, the world's first fairness as a service company. Financial institutions use FairPlay's APIs to embed fairness considerations into their marketing, underwriting, Pricing and collection algorithms as well as to automate their fair lending compliance. Previously, Kareem served as executive vice president at Zest AI, where he led business development for the company's machine learning powered credit underwriting platform. Prior to Zest AI, Kareem served as an executive at Softcard, a mobile payment startup that was required by Google. Kareem also served in the Obama administration. First as chief of staff to the State Department Special Envoy Envoy, excuse me, for climate change, where he helped manage the 50 person team that negotiated the Paris Climate Agreement. I wish I could do another podcast, Kareem, because I would love to talk about that. He was then senior advisor to the CEO of the Overseas Private Investment. Corporation OPEC, not to be confused with OPEC, uh, where he helped direct the U. S. government's 30 billion portfolio of emerging market investments with responsibility for transaction teams in Europe, Latin America, and in the Middle East. Kareem is a Forbes contributor and a frequent speaker on the application of AI to financial services. He is a graduate of Georgetown University Law Center with an honors graduate of the University of Chicago. Kareem, to say that I'm thrilled for you to be on this podcast is an understatement. Thank you so much. What a resume. What a resume. I am a lucky girl today. I'm a lucky girl. So let's talk about fairness because that is, that is your entire belly wick right now, but, and let's talk about fair play first. I talk a little bit about its mission, what it does, and I love the. Fairness as a service because everything has become a service now, but why not have fairness as well. So Take it away. Well, well, thanks for having me joanne. I'm absolutely delighted to be here um, I have been interested in the problem of Underwriting hard to score borrowers my whole career Uh, I got started doing that work in frontier emerging markets like sub saharan africa Eastern Europe, Latin America, the Caribbean. Uh, and that gave me visibility into the underwriting practices of some of the most prestigious financial institutions in the world. And what I was quite surprised to find is that. Even at the commanding heights of global finance, the underwriting methodologies, at least by Silicon Valley standards are still quite primitive, largely 20 to 50 variables and models built using old statistical techniques in Excel spreadsheets. Uh, but not only not only that, but I also found that almost all of the algorithms being used for credit underwriting and pricing and other high stakes decisions in financial services exhibit disparities towards people of color towards women towards other historically disadvantaged groups, by the way, that's not because the people who make those models are people of bad faith, right? Largely due to limitations in data and mathematics. But, you know, to account for those disparities, the industry, I think, has, um, you know, because it was lacking good tools to do better by historically disadvantaged groups, it kind of got in this mode of calling fancy consulting firms. And God forbid fancy law firms to come up with clever statistical and legal justifications for those disparities, rather than being able to actually enhance the fairness of those algorithmic systems. Uh, and so the good news is that the last several years have seen the emergence of new AI fairness methodologies, which do a better job of assessing the riskiness of populations that are not well represented in the data. Uh, and it turns out that the application of those AI fairness technologies to consumer credit models to consumer credit pricing models and other high stakes decisions in the customer journey can yield really great outcomes for folks who have been historically underserved. So we launched the company, and as you noted, we cheekily refer to ourselves as the world's first fairness as a service company, and our products allow anybody using an algorithm to make a high stakes decision about Someone's life to answer five questions. Is my algorithm fair? If not, why not? Could it be fairer? What's the economic impact to my business of being fairer? And finally, did we give our declines, the folks we rejected? A second look to see if we might, uh, have said no to someone we ought to have approved. So can you give me a little bit of example of how the platform works? You know, I use a case study or use case, they say. Sure. Well, so, um, what we find is that. Oftentimes there are variables that are being used to assess someone's riskiness, which may be appropriate for one group, but not for another group. And let me give you an example of that. So a variable that we encounter often in credit models is, uh, what is the applicant's employment? Right. And on the one hand, you might think about that and say, well, Kareem, consistency of employment is a totally, uh, it's totally related to creditworthiness. It's a, it has a very, very clear relationship to whether or not someone is going to pay back a loan. And I agree. Consistency of employment is a perfectly fine variable on which to assess the creditworthiness of a man. But consistency of employment All things being equal is necessarily going to discriminate against women between the ages of, say, 18 and 45, who take time out of the workforce to start a family. Uh, so part of the way that our system works is we expose our algorithms. during model development to the fact that, hey, algorithm, you will encounter a population in the world called women and women will sometimes exhibit inconsistent employment. And so before you deny an applicant for inconsistent employment, run a check to see if that applicant resembles good borrowers. On other dimensions, uh, that maybe your primary decisioning system didn't heavily take into account. And so by teaching the algorithm that there are these populations that are not well represented in the data and that, um, and because they are not well represented in the data, they may differ or exhibit different. Properties or characteristics, uh, than folks that the algorithm is used to encountering. We can make those decisioning systems more sensitive to historically disadvantaged groups. Interesting. So you're really peeling back the curtain a little bit about with, with respect to algorithms, because we all kind of see, you algorithm is. That's not my job, but you're, it seems to me and tell me if I'm, if I'm, if I'm correct. Your algorithms are not always set in stone. You're always going to be challenging your own algorithms. I think that's right. Because, you know, um, algorithms are just math that are used to make predictions. And the truth is for all of you. artificials, artificial intelligence and all of machine machines ability to learn. We find that those machines are capable of learning the wrong things. Let me give you one example. Sure. I first got started in this business. We I was working for a lender and we were trying to apply complex machine learning algorithms to build our credit. underwriting model. And in the early days of doing our testing of our algorithm, the algorithm came back and said to us, you should really make loans in Arkansas. And that was a very puzzling outcome to us because it just so happened to be that we knew that the regulatory environment in Arkansas was extremely hostile to the kind of loan that we were proposing. To make. Right. And so when we dug into the algorithm's reasoning a little bit, we found that the algorithm had, uh, had that the training set on which we used to build the algorithm did not include any loans from Arkansas. And so the algorithm concluded that loans never went. The data. Uh, is, is bad in our, that's so funny. That we should go make a bunch of lines there. And so machine learning algorithms are capable of learning the wrong things. And if you don't, um, use them with, uh, An acknowledgement. Upfront, uh, that these systems left to their own devices. Uh, could run your business off the rails. You're not going to ask the hard questions about whether their judgments can be relied upon. under conditions of stress, uh, or under conditions where something in the world has changed on DSO. One of the one of the key. Um, I think one of the key factors that anybody using an algorithm to make a high stakes decision about someone's life has to grapple with is what variables are these algorithms taking into account and to what extent Is that appropriate for all groups, not just a dominant group? And how is that algorithm reasoning going to change if there's a change either in the knock on door population or elsewhere in the macro environment, are you going to be able to rely on its recommendations? Building the systems to kind of govern and harness those algorithms and to have visibility into their reasoning and then also recourse to fix them if their performance degrades, is going to be key to successfully harnessing these technologies for good in the future. So when do you say you've harnessed enough or is it you never do? The work is never done, Joanne. Uh, you have to, you have to be monitoring these systems in production. And, uh, I can tell you, one of the things that I'm very concerned about right now, as, uh, you and the audience is, uh, undoubtedly aware, we have seen a dramatic rise in interest rates, uh, over the course of the last few months. Uh, that, you know, historically, when you've seen a rise in interest rates, that has had an effect. on consumer credit. Uh, at the same time, you know, we had all of these government moratoriums as a result of the pandemic, which prohibited negative credit reporting, right? So, so algorithms that are built on data from the last two years, uh, most assuredly have a kind of, um, inaccurate view. Well, they've expired. You know what, in my opinion, they've expired, you know, they're not valid anymore. Uh, and and they are likely to be challenged by a rising interest rate environment that is going to affect even those folks that might have looked good from a perspective of, you know, a year or two ago in the current environment. Right, right. So, I mean, we talked about accuracy of the algorithms. How do we decide or know if we reach the fairness threshold? Yeah. Okay. Can we ever? Yeah. Well, you know, the challenge there, Joanne, is that there are many different definitions of fairness. That's right. And in many cases, at least in financial services, the regulators have taken great care never to articulate what their preferred definition of fairness is. And then even if you can get folks to agree on a definition of fairness, getting them to agree on a threshold for what is considered fair or not has always been, is, you know, the subject of great debate. And so. Uh, I think that, you know, one of the challenges we face as an industry is coalescing, uh, with the regulators around a reasonable set of fairness definitions, uh, and thresholds. Uh, and by the way, that is like super contentious, uh, because some folks want to say, okay, well, they want to look at, you know, approval rates at what rate did you approve one group relative to another? Right. And then some folks want to look at, well, uh, what rate did you deny one group relative to another? And then how you define a group is also super complicated, right? Because, you know, um, regulators will sometimes say, well, we want to define groups the way that, uh, they, uh, the state sees them. So black Americans, Hispanic Americans, female Americans, but, um, but lenders will say, well, no, no, no. We want to define the group as people who are similarly situated from a risk perspective. Uh, and so these are all these are all very reasonable questions and debates to be had. Uh, but I think that there has not been sufficient formalism around, uh, sufficient grounding in what these definitions are and what the thresholds are. And as a result, I have a lot of sympathy for folks in the financial services space that are trying to be fair. That want to be fair, but that don't necessarily know what standards to hold themselves to. That's right. That's exactly right. I mean, I always hear it. You know, well, I treat everybody the same. That may be. But fairness really is a results based analysis. It's not an intention analysis, especially in financial services. So so fascinating. So speaking of regulators, um, you know, As you know, I, I, I, I talk a lot and I work a lot with with industry members who are subject to CFPB jurisdiction. And as we talked about before the podcast, you know, the CFPB's mission is to protect consumers and and and. The current director, Rohit Chopra, is laser focused on fairness right now and preventing discrimination. Um, and you're seeing it especially through data and algorithms, and you're seeing it in everything that they touch right now. Um, so a few weeks ago, uh, the CFPB issued an interpretive rule with respect to how service providers, which I'm going to tell you that Fair Play is a service provider, no doubt, um, how they deliver content. Um, in order to, in fact, affect consumer engagement, and it really was as we as we discussed a game changer, because now I don't see the CFPB in its rule. Try to interpret. Well, there's this kind of group and that kind of group to me. It's all 1. I don't see how they're going to make a distinction. But the idea now of using algorithms and delivering data. To target consumers for specific reasons. In the Bureau's mind could violate what we call the Consumer Financial Protection Act or be a UDAP. But I think that's going to be hard for companies like yourself and certainly the companies that you service. So I'm really interested to hear your thoughts on that because I think it's going to change a lot about how we see data being used. I agree with you. Look, I mean, I think historically, uh, the decisions in the customer journey that were most commonly tested for fairness where the underwriting decision and the pricing decision right in your and in your world, less so, but still to some extent, uh, you know, loss mitigation decisions. But now, now what we're seeing is. An expansion of the steps in the customer journey that have to be tested for fairness. And you're right. The regulators seem very focused on marketing fairness and the issues that arise out of personalization. But we're also seeing fraud, you know, what fairness and fraud screens, uh, is another area of great interest, uh, account management decisions, things like line assignment is another high stakes decision that needs to be tested for fairness. Uh, and then You know, headed into a choppy credit environment. I expect that things like loan modifications are going to have to be, uh, fairness. And so we're seeing a broadening of the kinds of decisions that need to be evaluated for fairness. And the kinds of players who operate across the customer journey, um, who might have to, you know, make decisions fairly on the behalf of the lender, on behalf of themselves or the lenders that they serve. And as you point out, uh, I think this most recent statement effect what I, what as I effectively refer to as marketing fairness, has sent shockwaves through the industry. I agree. Uh, we're, we're seeing it in our business. And I'll tell you, I don't think it's not an easy question at all. I was talking with one of our advisors who themselves is a very senior former regulator who enjoys the PBS news hour. And he was saying, you know, I don't know for a fact, but I'm guessing that the audience for the PBS news hour skews white. Uh, and so am I going to tell. And so I'm not sure that they can't advertise on the PBS news hour because that's a disparate impact issue that seems to me to be a somewhat absurd result. Uh at the same time, we do have a history of redlining right and Stewart on D. And geographic fairness. You know, not marketing in certain geographies. And so On the one hand, you can understand the risks that the regulators perceive as a result of digital marketing, especially in a world where seemingly fair variables are interacting in ways that encode race. So you might like build a marketing model and all the variables might look reasonable, but they might be interacting in ways that actually, uh, end up being proxies. And by the way, we can talk about how that happens. And I've got a kind of funny story, a cool story there to tell. But, um, but so, so on the one hand, you can sort of understand where the regulators are coming, uh, on this issue. On the other hand, once you dig into the details, uh, it's really, really hard to understand. The fairness of a marketing channel, uh, the fairness of advertising distribution, uh, et cetera. Yeah, I, I agree. I mean, I, the thought that came to my mind is any financial institution that has a product offer. I mean, they're offering the product to their existing consumer base, but of course they're going to target, you know, I was saying to someone today, you know, we're back to school. So, you know, do you want to, do you need some money for back to school? Well, you know. More than likely they're going to target people who don't have a lot of income and could use the credit to help their kids get some books. Are you targeting the wrong way? I think it's, it's very blurry. Very, very blurry. I think the, I understand the intent, but we're all getting marketed. I mean, you're going to get off this call and you're going to look at your Instagram and you're being marketed. You're being targeted. Because of who you are. So very, very scary. Um, I think where there's going to be a lot of hard thinking for folks like you to do, uh, Joanne is, you know, finding the disparities in some sense is easy. The question will be, are the disparities justified? And how do you think about a legitimate business necessity, uh, in a context like the one that we're talking about? Uh, and, and there, I think more work More thinking has to be done on the part of industry and on the part of the regulators. And I hope that we can have a dialogue about that, that lands us in a reasonable place, right? And the efficiencies of all of it. I mean, we're all doing this to be efficient. It seems to me that this is kind of a put on the brakes moment and we've got to keep, you know, looking back and looking back, and then it's inefficiencies, which. You know, inefficiencies are going to mean that costs are going to rise. That's, that's ultimately, that's what's going to happen. So very interesting. So, you know, my last point I want to talk to you about, and you've hinted in this, in our conversation. So this idea of fair lending principles, as we're seeing now, we're going to look at marketing. Um, as you pointed out, you know, I've been in the debt collection space for, you know, longer than I, that I will admit on, on, on air. But, um. When the CFPB came out several months ago, earlier in the year, they had an advisory opinion about fair lending principles throughout the entire credit cycle. And if you look at ECOA and you look at the definition of a credit transaction, it is from origination to collection. It's the whole thing. The collection doesn't think about it that way. They think about it when we're getting it at the tail end. But I think Chopra's right. I mean, under the definition, It's got to encompass everything. But boy, that advisory opinion really scared a lot of people in the industry that I know, because that is not something that they have ever thought about. Now, we've seen a lot of articles and studies, you know, why poor neighborhoods are getting sued more. And I get all of that. But a lot of my clients say to me, where do I begin with this? How do I even start to think about and look at the data that I am collecting and figuring out whether my collection efforts are being fair? I that is not in their psyche. Yeah. Any advice , uh, yeah, call My advice is call fair or play. Um, yeah. Uh, besides that, um, sure. Well look, I think that, um, Uh, sunlight is the best disinfectant, right? You know, even though some sunlight, sometimes you get burned by sunlight, but you got to put some sunscreen on. Yeah, exactly right. And so, so I, you know, I think the place to start and by the way, and I have sympathy for folks like your customers because they don't have a lot of experience doing this work. Like you don't even know in many cases, which. You know, which defaulted borrower is Hispanic, which defaulted borrower black, right? So, so one place to start is by simply doing that demographic imputation, right? Figuring out, okay, uh, I've just bought this portfolio of, uh, defaulted, defaulted debt. What is the demographic composition of that portfolio? Uh, and then there are some very basic. Kind of tests that you can compute to understand, okay, is one group experiencing a positive outcome at a higher, lower rate than another group. So is one is one group being, you know, foreclosed on at a higher rate is one group being offered. Loan mods that are more generous than another group are, you know, there's one group getting its calls returned or not returned at a higher or lower rate than another group. The first step is transparency into the demographic membership of your portfolio. And I acknowledge that that's hard because you don't have that data. Uh, and then, and then trying to assess, uh, whether or not certain groups might be experiencing positive outcomes at higher, lower rates than other groups, uh, and, and if so, why, and that's got to be the starting point. For the inquiry. Uh, and I acknowledge it is without a Sherpa or without good technology. You're gonna have a lot of trouble with that. I agree. Well, as you said in our conversation before, uh, fairness is awareness. So it's now going to be awareness, but it's, you know, you raised what you just said made me think, um, knowing the demographics of your group, that's not something, you know, I know from the, in the debt buying industry, which I have some. Thank you. Relationship with but that those aren't things that you think about. And so now I'm wondering if we have to kind of know that how that's going to impact that market, you know, will will portfolios and from poor neighborhoods now fetch a higher price because you're lower price because you're going to have to work them harder than you would. From some other type of demographic. So it really, it's going to be fascinating how all this is going to play out. I don't know how it's going to play out, but we're like in the top of the first inning. Yeah, seriously. Seriously. I don't think we've even gotten to the ballpark yet. I'm trying to look for a parking space at this point. Oh, God. Cream. What a great discussion. Thank you so much for sharing your insight on this. I think more to come. Absolutely more to come on this. So I really appreciate it. Um, but before I let you go, um, as I said, I always ask to, I asked my guest two questions, uh, in this podcast. So I hope you'll, you'll play along as well. Uh, the first question I ask is, uh, So when I started the podcast, uh, it was in the middle of COVID and we were all sitting at home. We're all still sitting at home, but now we can go to the grocery store without a mask. So that's, that's the difference. Um, but so I would ask people, you know, what are you doing while you're at home? Got a wonderful responses, but now that we're, you know, hopefully getting back to normal, whatever that means. My question is, what are you doing now that you wouldn't have done? But for COVID. Yeah. What am I doing now that I would not have done but for COVID? Um, you know, we, uh, we have just like resolved to go out more, you know, uh, to muse live music, comedy shows, you know, live performances. You sort of forget how much you take that stuff for granted, uh, until you've been shut away for two years. That's exactly right. That's it. Right. So seeing your neighbors, we'll call it that experiencing going out within the community. I love it. I love it. Well, thank you. Um, second thing I asked all my guests is, uh, when I did start the podcast, I did it as a little bit of a food theme because, uh, Watching TV and seeing people in car lines for food in this country was pretty shocking to me. I had never seen that before, you know, and that really resonated a lot with me. So when I started to do the podcast, I would ask guests, you have a food bank in your neighborhood or, you know, something that, uh, is, you know, helping people throughout COVID. And I got wonderful responses and I learned about. Some really fascinating organizations. Now, of course, it's any organization that you and your lovely wife, Melissa, have a, uh, have an affinity for and you'd like to shout out and the podcast will make a small donation on your behalf. Oh, that's that's absolutely wonderful, Joanne. Well, we really love the work being done by Covenant House. We're very kind of Covenant House. I believe exists in many different cities. It does. It does. Yeah. So we absolutely love the work done by Covenant House to provide shelter for homeless youth. Um, that's a real, I live in LA and that's a real problem. Here in L. A. And I just think that their approach is humane and rational and does a really great job of getting, um, you know, shelter over the heads of kids that need it and then helping as much as they can to equip them for, you know, life in what can sometimes be a rough world. I agree. I agree. Wonderful. Well, we will absolutely reach out to them and happy to make that donation. So Kareem, thank you again so much for coming onto the podcast and many thanks to our credit eco to go listeners for tuning in and logging on. All episodes of credit to go to go can be found on buzzsprout and Spotify, as well as apple podcasts. And I believe odyssey, um, information on our podcast can be found on my clarkhill. com bio page, as well as on my LinkedIn page. If you'd like to be a guest on the show or have ideas for future show topics, please email us at credit ego to go at clarkhill. com. Thank you. Be well and stay safe. This podcast is intended for general education and informational purposes only and should not be regarded as either legal advice. or a legal opinion. You should not act upon or use this publication or any of its contents for any specific situation. Recipients are cautioned to obtain legal advice from their legal counsel with respect to any decision or course of action contemplated in a specific situation. Clark Hill PLC and its attorneys provide legal advice only after establishing an attorney client relationship through a written attorney client engagement agreement. This recording does not establish an attorney client relationship with any recipient.